Predictive maintenance technologies of lasers in Huaris Laser Cloud (HLC)
Predictive maintenance technologies has emerged as a groundbreaking approach in various industries, ensuring optimal equipment performance, reducing downtime, and ultimately saving costs. In this article, we delve into the world of predictive maintenance with a specific focus on laser systems. Join us as we explore the concepts, technical intricacies, and the remarkable business analysis within Huaris Laser Cloud (HLC).
Predictive maintenance technologies, powered by cutting-edge technology, is transforming the way we manage laser systems. Huaris Laser Cloud, at the forefront of this innovation, offers a game-changing solution. In this article, we will not only demystify the predictive maintenance concept but also unravel the technical aspects that make HLC an industry leader. Moreover, we will delve into the economic impact of adopting such an advanced system for laser maintenance. Stay with us as we journey into the future of laser system management with predictive maintenance technologies and Huaris Laser Cloud.
In this article you will learn:
Preventive and predictive maintenance technologies in manufacturing
Preventive maintenance technologies are based on a regular maintenance schedule that is designed to identify and correct potential problems before they lead to a failure or a significant reduction in the performance of the laser. Preventive maintenance technologies can include tasks such as cleaning and aligning the laser’s optics, replacing worn or damaged components, and performing calibration, alignment, cleaning and performance tests.
Predictive maintenance technologies are based on more advanced maintenance strategy that uses data and analysis to predict when a failure or a significant reduction in performance is likely to occur, and schedule maintenance accordingly. Predictive maintenance technologies can include tasks such as monitoring the laser’s power, beam width, and pointing stability, as well as analyzing data from the laser’s control systems to detect patterns or trends that may indicate a problem with the laser.
Differences between preventive and predictive maintenance technologies

Preventive maintenance of lasers
Preventive maintenance tools schedule is typically created based on the historical data collected over some period of time and the user learns from this data about the laser performance. Normally the schedule is periodic. Based on the data the typical failure periods are calculated and the schedule for maintenance is set with the periodicity of maintenance actions occurring before the failure is expected.
Predictive maintenance of lasers
The predictive maintenance technologies, on the other hand, is normally implemented based on the analysis of the life data where various trends and events are monitored. Based on the collected information the critical parameters are extrapolated in time and from this extrapolation the malfunction is predicted to advise on certain actions to be taken only before they are expected. This approach implies that the maintenance actions are performed on demand and not on the periodic basis, as in the technologies of preventive maintenance .
Preventive and predictive maintenance concepts have been briefly discussed in one of our previous articles here: Preventive and predictive maintenance of lasers.
Predictive maintenance for manufacturing - comparison of various maintenance models
Very diverse approaches to maintenance could be found in practice. Generally speaking, in the predictive maintenance for manufacturing, people either perform preventive maintenance or they just repair the equipment as soon as it gets broken.
The latter model is very simple in concept: when a device or machine gets broken, the maintenance team diagnoses the source of malfunction, parts are ordered, replaced and then the work could be resumed. This model though has very serious implications.

Some of them are: the downtime of the device or machine could be very long because the procurement process starts after the failure is detected and diagnosed. Moreover, the delivery also could have a long lead time which also adds up to the total length of a pause of the work. Not diving too deeply into the consequences of this model, it has to be noted that even though the cash is not frozen in the spare parts stored in the depo, the reduction of income from the loss of production capacity due to the long downtime has to be seriously considered.
A simple use case analysis showing the significance of this problem.
On the other hand, the deployment of the preventive maintenance technologies model induces several business processes. Eg. it assumes that the certain procurement processes have to be conducted on the regular basis in the very right moment of time to assure the spare parts are available anytime the maintenance work needs to be done. Normally tasks of the preventive maintenance tools are well defined and thus manageable. For instance, it is very clear how much time it takes to replace some worn component. Thus the machine productivity could be well calculated and maintenance can be planned in the proper way to minimize interference with the device users. Moreover, typically this model implies that the downtime is strongly reduced when compared to the repair-when-broken model, the productivity increased and, in the case of the business organizations, the revenue improved.
The predictive maintenance technologies model obviously brings even more benefits than the preventive maintenance because it reduces the scope of maintenance and the capital “frozen” in the spare parts procurement process. However, for its proper operation it assumes availability of significant amounts of data which is not always possible.
Laser downtime cost - case study analysis
As already elaborated, downtime means losses. Be it financial if you are in a company or it might mean it is loss of beam time at a hardly accessible, expensive research apparatus. Let’s make a quick intellectual exercise to present the importance of the problem. Our case study is a laser system used in a beauty clinic specialized in the scar removal procedure.
Here are assumptions:
- Cost of a laser system: 50 000 EUR
- Depreciation rate: 30%
- Monthly depreciation value: 1 250 EUR
- Cost of a single visit of a patient: 100 EUR
- Time of a single treatment: 15 min
- Work time a day: 8 hours
- Income a day: 100*(60/15)*8 = 3 200 EUR
- Income a week: 5 days * 3 200 EUR = 16 000 EUR
- Income over 5 weeks: 5 * 16 000 EUR = 80 000 EUR
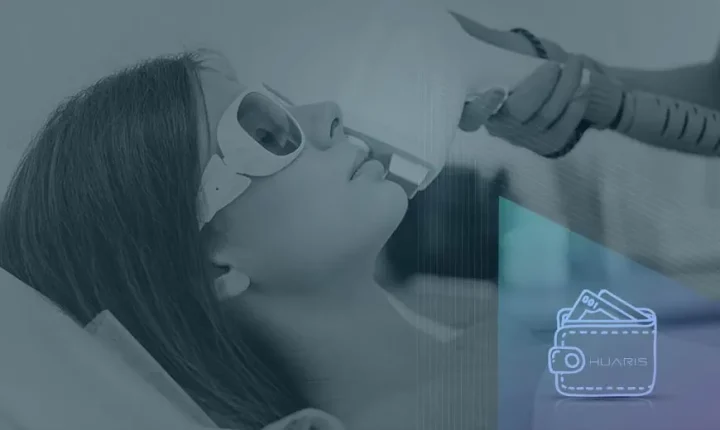
(Photo from Freepick)
Frequently occurring downtime is 4-6 weeks. Thus we assumed an average 5-weeks. According to the estimations presented above the total losses of a clinic working only with 1 laser during a 5-week period are: 80 000 EUR + 1 250 EUR = 81 250 EUR! These include only ownership costs related to the lost income and depreciation. The cost of the repair service and spare parts could fluctuate a lot so we are not adding that sum here but it has to be considered, too.
If now the predictive maintenance action is taken then typically the downtime is reduced to a few hours preventive work. If we assume:
- Preventive maintenance work time: 5 hours
- Total loss of income: 100*(60/15)*5 = 2 000 EUR
- Depreciation during 5 hours is assumed: 650 EUR
- Total loss: 2000 + 650 = 2 650 EUR
In this scenario the cost of maintenance is also not considered as it could be very different from one case to another but typically it is much lower in the latter case.
Obviously this is only a demo exercise and the benefits from implementing the predictive maintenance in your organization with your laser will have to be estimated specifically but it is very clear that the gain could be very significant and the predictive maintenance is definitely worth deploying.
Maintenance problem in lasers: status quo
Preventive and predictive maintenance models are a golden standard in industrial equipment. However, for various reasons, it has not been implemented in the laser systems so far. Due to this fact, most of the time, the companies and research institutions work in the most primitive model of repair-when-broken.
It is worth considering why the lasers have no predictive maintenance available. The simple answer to this question is: so far there were no tools to do that! As mentioned earlier, the predictive maintenance model assumes collection and processing of significant amounts of data and only over the recent years this became possible.
As there were no tools allowing deployment of the predictive maintenance in the laser systems, up to now, the laser systems had no option but to get a repair after they got broken. This essentially meant that the downtimes weeks or even months long are well known to the people from the field.
Also the fact is that quantitative data about laser system performance in the long term has not been available. For this reason the various instabilities of the lasers have been known and observed but they were not measured in the long term. And, as every manager knows, if something is not measured, it is not managed. It could be very teaching if the laser behavior is analyzed over a long period of time.
As an example the measurement results of the laser beam width are presented in the graphs below. The graphs present roughly 1-month perspective.
Such significant fluctuations will impact the laser beam interaction with the target. Regardless the application: metal sheet cutting or plasma creation and research because e.g. the increase of the laser beam width imply the increase of the area over which the intensity is distributed. Consequently, the interaction of the beam with the matter is compromised.
The above presented data come from the measurement of real laser systems at the production site of Perspectiva Solutions.
How Huaris Laser Cloud deploys predictive maintenance concept?
Huaris Laser Cloud (HLC) system is the first, advanced and universal tool for the deployment of predictive maintenance technologies in the laser systems.
The general architecture has a few key pillars:
- The laser beam is monitored by the dedicated sensor in the industrial solutions or by the laser beam profiler in other applications. Optionally a separate power meter may be added.
- The sensor is connected to a local PC or a dedicated tablet (Mobi version of Huaris laser beam profilers).
- Locally, the data is preprocessed and some scope of information is displayed for instant viewing.
- The local application is also responsible for the communication with the remote database.
- Artificial intelligence (AI) algorithms fully automatically analyze streamed data in the real time and render the results to the laser owner and, optionally, to a maintenance company.
- The web application presents the historical and live data, displays notifications with the laser malfunction discoveries and recommendations and allows user management
Some important features include:
- The web application allows remote laser management
- Web application is system-diagnostic: it can be used on Mac, Windows, tablet or smartphone.
- Artificial intelligence models analyze the data instantly and automatically
- The web application is common to individual laser beam profilers, power meters and dedicated industrial sensors. In other words: one solution for all clients.
- AI components have pre-trained models which are common for all lasers, however, they are also periodically re-trained to consider each laser specificity.
- AI models analyze not only the laser beam quality in a given moment of time but also their change over some period of time (trends analysis) giving a clear indication about laser performance historically and in the future!
- Web application generates notifications and suggestions displayed within the application, sent by email or SMS.
Few example artifacts detected by artificial intelligence in the HLC

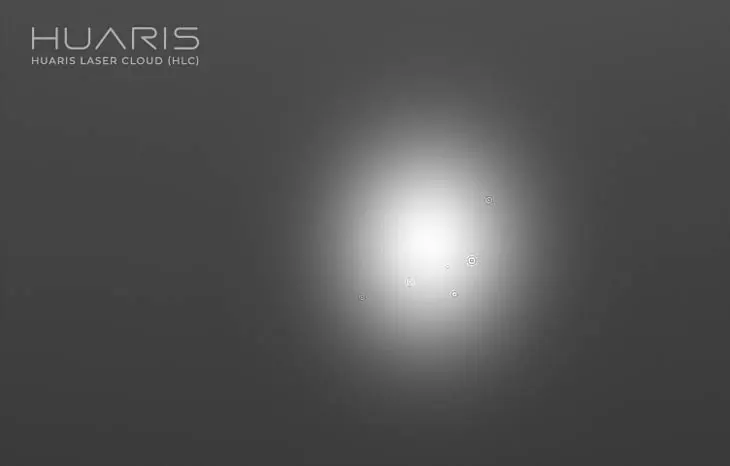
Dust - Example artifact detected by AI in the Huaris Laser cloud(HLC)


Linear diffraction example artifact detected by Huaris Laser Cloud (HLC)

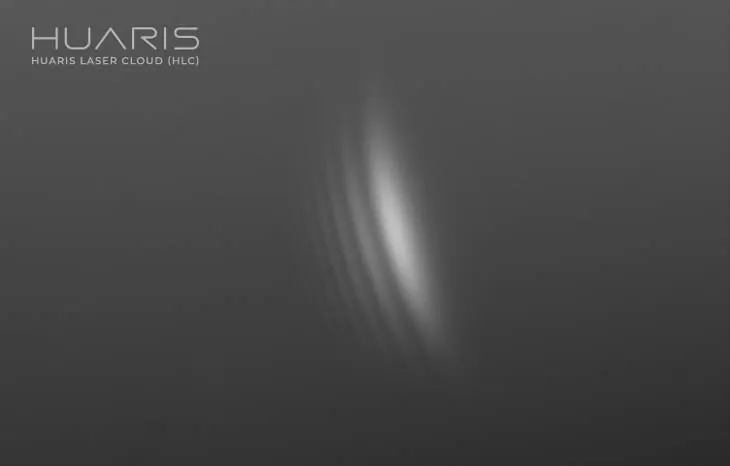
Diffraction on rounded structure example artifact detected by HLC


Spherical artifact detected by Huaris Laser Cloud - (HLC)
Where is it applicable?
HLC is applicable in a very broad range of products and its applicability is determined primarily by the laser wavelength. Generally speaking, though, it can be deployed in very different lasers and these very different lasers could be managed from the same web application.
See our video tutorials
Key takeaways
The deployment of the predictive maintenance methodology in laser systems brings numerous benefits. They include

For the laser owner:
- Cost reduction
- Increase of laser availability
- Increase of revenues
- Remote management of the laser systems
- Automated diagnostics
- Automated notifications and maintenance recommendations
For maintenance company:
- Tool for the management of laser maintenance tasks
- Tool suggesting maintenance actions
- Automated diagnostics
- Automated notifications and maintenance recommendations
Final note: Huaris is a brand by Perspectiva Solutions Ltd. HLC is a proprietary technology of Perspectiva Solutions Ltd.
Recent posts about laser beam profiler
Author